|
ACCESS THE FULL ARTICLE
No SPIE Account? Create one
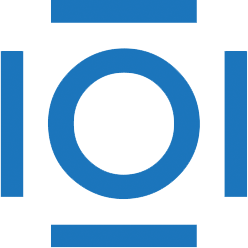
CITATIONS
Cited by 2 scholarly publications.
Principal component analysis
Independent component analysis
Statistical analysis
Sensors
Minerals
Interference (communication)
Binary data