|
ACCESS THE FULL ARTICLE
No SPIE Account? Create one
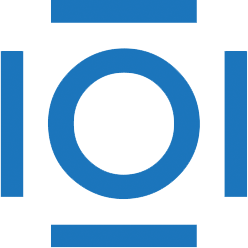
CITATIONS
Cited by 1 scholarly publication.
Monte Carlo methods
Pattern recognition
Mahalanobis distance
Statistical analysis
Distance measurement
Modeling
Physics