|
ACCESS THE FULL ARTICLE
No SPIE Account? Create one
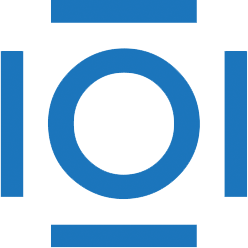
CITATIONS
Cited by 1 scholarly publication.
Machine learning
Sensors
Signal detection
Liquids
Signal processing
Feature extraction
Solids