|
ACCESS THE FULL ARTICLE
No SPIE Account? Create one
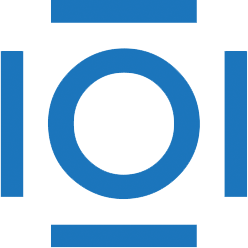
CITATIONS
Cited by 217 scholarly publications.
Algorithm development
Tolerancing
Radon
Matrices
Reconstruction algorithms
Algorithms
Compressed sensing