|
ACCESS THE FULL ARTICLE
No SPIE Account? Create one
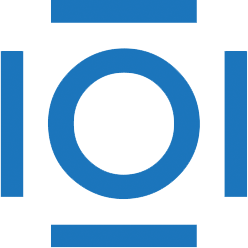
CITATIONS
Cited by 8 scholarly publications.
Machine learning
Visualization
Neural networks
Automatic target recognition
Computer programming
Data modeling
Sensors