|
ACCESS THE FULL ARTICLE
No SPIE Account? Create one
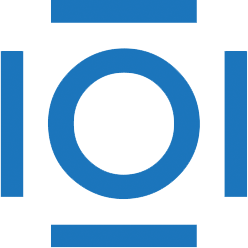
CITATIONS
Cited by 35 scholarly publications.
Hyperspectral imaging
Image classification
Image fusion
Matrices
Distance measurement
Neptunium
Spatial analysis