|
ACCESS THE FULL ARTICLE
No SPIE Account? Create one
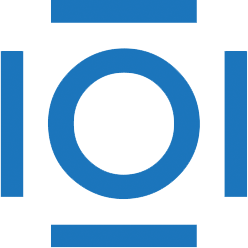
CITATIONS
Cited by 1 scholarly publication.
Hyperspectral imaging
Image analysis
Target detection
Error analysis
Feature extraction
Image quality
Remote sensing