|
ACCESS THE FULL ARTICLE
No SPIE Account? Create one
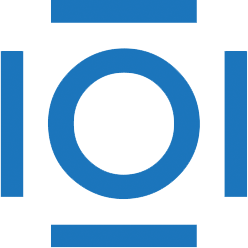
CITATIONS
Cited by 3 scholarly publications.
Magnetic resonance imaging
Computed tomography
X-ray computed tomography
Image restoration
CT reconstruction
Scanners
Fourier transforms