|
ACCESS THE FULL ARTICLE
No SPIE Account? Create one
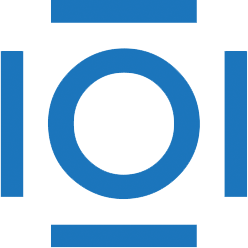
CITATIONS
Cited by 3 scholarly publications and 2 patents.
Databases
Image quality
Associative arrays
3D image processing
Rutherfordium
3D modeling
Visualization