|
ACCESS THE FULL ARTICLE
No SPIE Account? Create one
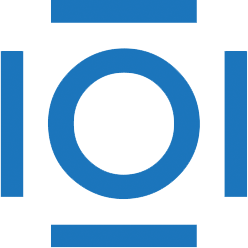
CITATIONS
Cited by 1 scholarly publication.
Particles
Particle filters
Nonlinear filtering
Filtering (signal processing)
Electronic filtering
Digital filtering
Monte Carlo methods