|
ACCESS THE FULL ARTICLE
No SPIE Account? Create one
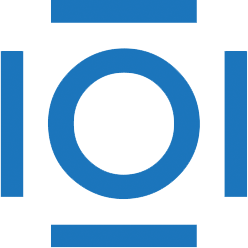
CITATIONS
Cited by 3 scholarly publications.
Associative arrays
Chemical species
3D image processing
Image processing
Computed tomography
Denoising
Medical imaging