|
ACCESS THE FULL ARTICLE
No SPIE Account? Create one
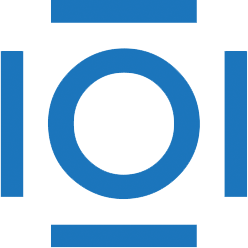
CITATIONS
Cited by 2 scholarly publications.
Image segmentation
Positron emission tomography
Tumors
Computed tomography
Lung
3D image processing
Spine