|
ACCESS THE FULL ARTICLE
No SPIE Account? Create one
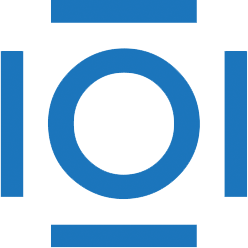
CITATIONS
Cited by 1 scholarly publication.
Bladder
Image segmentation
Bladder cancer
Feature extraction
Computing systems
Computer aided design
Convolutional neural networks