|
ACCESS THE FULL ARTICLE
No SPIE Account? Create one
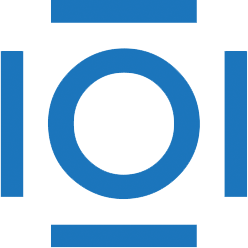
CITATIONS
Cited by 4 scholarly publications.
Image segmentation
Associative arrays
Tissues
Pathology
Image processing algorithms and systems
Image resolution
Cancer