|
ACCESS THE FULL ARTICLE
No SPIE Account? Create one
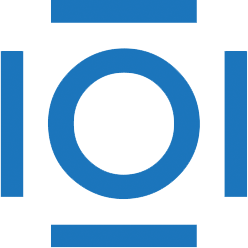
CITATIONS
Cited by 10 scholarly publications.
Doppler effect
Convolution
Radar
Convolutional neural networks
Breast
Detection and tracking algorithms
Machine learning