|
ACCESS THE FULL ARTICLE
No SPIE Account? Create one
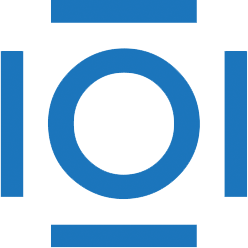
CITATIONS
Cited by 2 scholarly publications.
Machine learning
Databases
Feature extraction
Ions
Neodymium
System identification
Web 2.0 technologies