|
ACCESS THE FULL ARTICLE
No SPIE Account? Create one
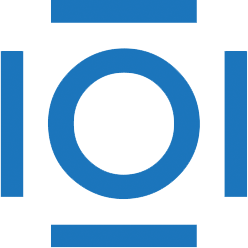
CITATIONS
Cited by 15 scholarly publications and 1 patent.
Signal to noise ratio
Super resolution
Deconvolution
Radar imaging
Radar
Antennas
Scattering