|
ACCESS THE FULL ARTICLE
No SPIE Account? Create one
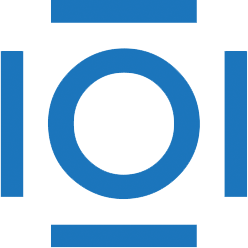
CITATIONS
Cited by 19 scholarly publications.
Data modeling
Near infrared
Reflectivity
Spectroscopy
Sensors
Agriculture
Principal component analysis