Object detection is one of the most important research topics for remote sensing image (RSI) analysis. Because of the rapid development of satellite imaging technology, the resolution of RSI has increased dramatically, which brings a great challenge to the efficiency of RSI-based object detection. We present an object detection model, which greatly improves the detection speed by using learning-based hashing techniques. Specifically, first, a selective search method is used to generate many high quality object proposals that may contain objects. Then for each proposal, we learn rotation-invariant binary codes, which can handle the objects with arbitrary orientations in RSI, to quickly eliminate most nonobject proposals in Hamming space. And finally, the object detection task can be achieved by classifying the left (very limited amount of) proposals with more discriminating classification model. Experimental evaluations on a public very high-resolution remote sensing dataset show the superiority of the proposed method. Specifically, the rotation-invariant learning metric has been combined with three popular hashing methods, and performance improvements have been obtained for all the cases. Furthermore, comparisons with deep learning-based detectors show that hash-based methods fail to achieve state-of-the-art detection performance, but when the graphics processing unit is inaccessible, it achieves a good compromise between performance and runtime. |
ACCESS THE FULL ARTICLE
No SPIE Account? Create one
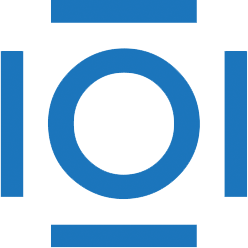
CITATIONS
Cited by 2 scholarly publications.
Binary data
Remote sensing
Sun
Image segmentation
Feature extraction
Sensors
Data modeling