In recent years, graph convolutional networks (GCNs) have attracted increased attention in hyperspectral image (HSI) classification through the utilization of data and their connection graph. However, most existing GCN-based methods have two main drawbacks. First, the constructed graph with pixel-level nodes loses many useful spatial information while high computational cost is required due to large graph size. Second, the joint spatial-spectral structure hidden in HSI are not fully explored for better neighbor correlation preservation, which limits the GCN to achieve promising performance on discriminative feature extraction. To address these problems, we propose a multiscale graph convolutional residual network (MSGCRN) for HSI classification. First, to explore the local spatial–spectral structure, superpixel segmentation is performed on the spectral principal component of HSI at different scales. Thus, the obtained multiscale superpixel areas can capture rich spatial texture division. Second, multiple superpixel-level subgraphs are constructed with adaptive weighted node aggregation, which not only effectively reduces the graph size, but also preserves local neighbor correlation in varying subgraph scales. Finally, a graph convolution residual network is designed for multiscale hierarchical features extraction, which are further integrated into the final discriminative features for HSI classification via a diffusion operation. Moreover, a mini-batch branch is adopted to the large-scale superpixel branch of MSGCRN to further reduce computational cost. Extensive experiments on three public HSI datasets demonstrate the advantages of our MSGCRN model compared to several cutting-edge approaches. |
ACCESS THE FULL ARTICLE
No SPIE Account? Create one
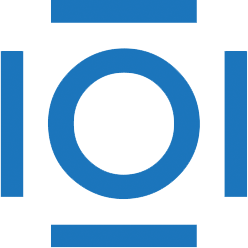
CITATIONS
Cited by 1 scholarly publication.
Convolution
Feature extraction
Hyperspectral imaging
Image segmentation
Education and training
Matrices
Data modeling