|
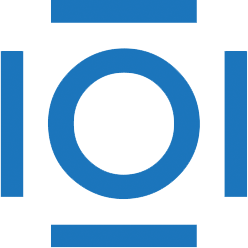
CITATIONS
Cited by 38 scholarly publications and 1 patent.
Confocal microscopy
Tissues
Ovarian cancer
Feature selection
Error analysis
Natural surfaces
Principal component analysis