|
ACCESS THE FULL ARTICLE
No SPIE Account? Create one
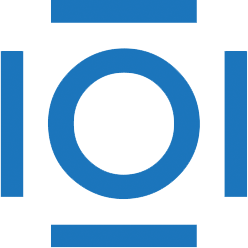
CITATIONS
Cited by 1 scholarly publication.
Image segmentation
Image processing algorithms and systems
Performance modeling
Process modeling
Data modeling
Lithium
Curium