|
ACCESS THE FULL ARTICLE
No SPIE Account? Create one
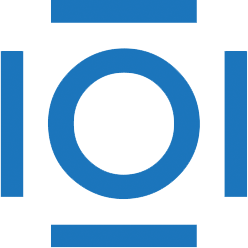
CITATIONS
Cited by 2 scholarly publications.
Image segmentation
Convolution
Roads
Sensors
Visualization
Dimension reduction
Intelligence systems