|
ACCESS THE FULL ARTICLE
No SPIE Account? Create one
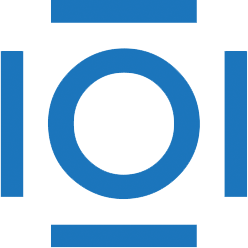
CITATIONS
Cited by 2 scholarly publications and 1 patent.
Video
Detection and tracking algorithms
Sensors
Motion models
Databases
Video processing
Cameras