|
ACCESS THE FULL ARTICLE
No SPIE Account? Create one
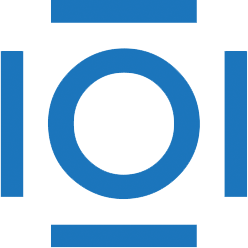
CITATIONS
Cited by 1 scholarly publication and 1 patent.
Air contamination
Network architectures
Image restoration
Visualization
Image transmission
Atmospheric modeling
Image fusion