We propose a saliency-enhanced two-stream convolutional network (SETNet) for no-reference image quality assessment. The proposed SETNet contains two subnetworks of image stream and saliency stream. The image stream focuses on the whole image content, while the saliency stream explicitly guides the network to learn spatial salient features that are more attractive to humans. In addition, the spatial attention module and dilated convolution-based channel attention module are employed to refine multiple levels features in spatial and channel dimensions. Finally, the image stream and saliency stream features fusion strategy is proposed to integrate features at the corresponding layer, and the final quality scores are predicted by using multiple levels of integrated features and weighting strategy. The experimental results of the proposed method and several representative methods on four synthetic distortion datasets and two real distortion datasets show that our SETNet has higher prediction accuracy and generalization ability. |
ACCESS THE FULL ARTICLE
No SPIE Account? Create one
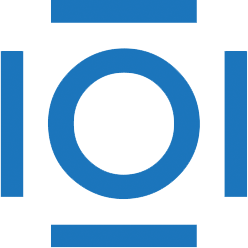
CITATIONS
Cited by 2 scholarly publications.
Image quality
Distortion
Image fusion
Image enhancement
Feature extraction
Convolution
Networks