In view of the difficulty in accurately recognizing vehicle models due to the wide variety of vehicle models and little differentiation between some models, a structure-guided attention network for weakly supervised learning fine-grained vehicle model recognition is proposed. First, we utilize the structure-guided attention location module to locate key features of images, extracting the strong and weak attention areas of the image by generating attention maps. Second, we investigate multichannel convolutional neural network for the learning of the strong-weak feature pairs and solve the problem of different network feature maps with different sizes by using feature map alignment. Third, an adaptive weighting method is proposed to balance the loss function. The experimental results show that our algorithm effectively improves the accuracy of the fine-grained vehicle recognition dataset and has universal applicability in other fine-grained recognition datasets. |
ACCESS THE FULL ARTICLE
No SPIE Account? Create one
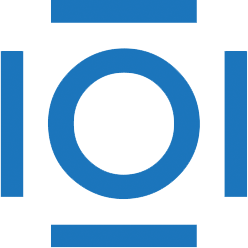
CITATIONS
Cited by 1 scholarly publication.
Detection and tracking algorithms
Feature extraction
Data modeling
Machine learning
Mirrors
Systems modeling
Performance modeling