The process of single-image super-resolution (SR) has certain limitations, such as an insufficient utilization of high-frequency information in images and a network structure that is insufficiently flexible to reconstruct the feature information of different complexities. Therefore, deep iterative residual back-projection networks are proposed. Residual learning was used to ease the difficulty in training and fully discover the feature information of the image, and a back-projection method was applied to study the interdependence between high- and low-resolution images. In addition, the network structure reconstructs smooth-feature and high-frequency information of the image separately and transmits only the residual features among all residual blocks of the network structure. The experiment results show that compared with most single-frame image SR methods, the proposed approach not only achieves a significant improvement in objective indicators, but it also provides richer texture information in the reconstructed predicted image. |
ACCESS THE FULL ARTICLE
No SPIE Account? Create one
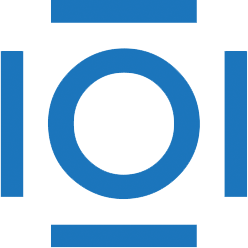
CITATIONS
Cited by 1 scholarly publication.
Super resolution
Lawrencium
Feature extraction
Performance modeling
Convolution
Reconstruction algorithms
Image processing