Few-shot learning (FSL) has been widely used for image classification when the training samples are limited and can effectively avoid overfitting. Most FSL methods are based on metric learning and tend to learn features that are conducive to classifying known classes of images. However, these methods cannot capture the semantic features that are important for classifying new classes of images. To address this issue, we proposed an improved few-shot image classification model based on multi-task learning termed SIM. It combines the self-supervised image representation learning task with the supervised image classification task, thus utilizing the complementarity of these two tasks. SIM has two stages, i.e., the pre-training stage and the meta-training stage. In pre-training stage, we learned the representation of training images via supervised learning and self-supervised learning. Then, in meta-training stage, we trained a linear classifier based on the learned representation. According to the experiments on four data sets, including three natural image data sets and one marine plankton image data set, SIM outperformed existing methods and achieved quite good performance in complex application scenarios. |
ACCESS THE FULL ARTICLE
No SPIE Account? Create one
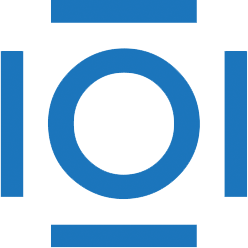
CITATIONS
Cited by 2 scholarly publications.
Image classification
Data modeling
Performance modeling
Machine learning
Statistical modeling
Solid state lighting
Ocean optics