Few-shot class-incremental learning (FSCIL) is crucial and practical for artificial intelligence in the real world, which learns novel classes incrementally from few samples without forgetting the previously learned classes. However, FSCIL confronts two significant challenges: “catastrophic forgetting” and “overfitting new.” We focus on convolutional neural network (CNN)-based FSCIL and propose a human cognition-inspired FSCIL method, in which the knowledge of novel classes is learned under the guidance of the previously learned knowledge. Specifically, we learn a discriminative and generalized CNN feature extractor from the base classes in the first task. We generate the representations of base and novel classes in unified feature space without training on novel classes, thus avoiding “forgetting old.” For the novel classes in long sequential tasks, beyond the representation generation, we enhance the representation by exploring the correlations with the previously learned classes to alleviate overfitting new and ensure that the novel classes adapt to the feature space. Experimental results show that our proposed method achieves very competitive results on MiniImageNet and CIFAR-100 datasets. |
ACCESS THE FULL ARTICLE
No SPIE Account? Create one
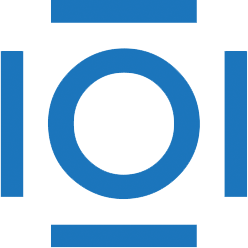
CITATIONS
Cited by 1 scholarly publication.
Data modeling
Prototyping
Fourier transforms
Visualization
Performance modeling
Artificial intelligence
Convolutional neural networks