1.IntroductionAdequate cerebral blood flow (CBF) ensures delivery of oxygen and required substrates to maintain normal brain function. Assessment of CBF can aid in the diagnosis and management of numerous conditions, including stroke and traumatic brain injury. Several techniques exist to assess CBF, including perfusion magnetic resonance imaging (MRI), computed tomography, and transcranial Doppler ultrasound. Drawbacks of these approaches include the need for patient transport, exposure to ionizing radiation, and/or lack or microvascular sensitivity. Diffuse correlation spectroscopy (DCS) is an emerging optical tool that quantifies an index of regional microvascular cerebral blood flow. This approach is especially well suited for continuous, beside monitoring,1,2 given its high temporal resolution ( to 100 Hz), use of non-ionizing radiation, relatively low cost (< $50 k), and portability. In DCS, near-infrared light is injected into the tissue surface and detected some distance away (typically 1 to 3 cm). Red blood cell motion induces temporal fluctuations in the detected light intensity.3 A simple analytical model is used to relate these intensity fluctuations to an index of blood flow (BFI, ) of the underlying tissue.4 However, by the nature of this noninvasive measurement, light must pass through extracerebral layers (i.e., skull, scalp, and/or cerebral spinal fluid) before detection at the tissue surface. Thus, the BFI measured by DCS reflects a combination of both cerebral and extracerebral hemodynamics. Multiple methods have been proposed to minimize extracerebral contributions to BFI and to improve brain sensitivity, including both hardware5–9 and novel analytical approaches.10,11 Analytical approaches are particularly attractive because they can be integrated into the analysis strategies of any hardware approach. Among those approaches, a model that treats the head as a series of three parallel, infinitely extending slabs (mimicking scalp, skull, and brain) has been shown to provide a significant improvement in CBF estimation over the traditional DCS analysis approach that treats the head as a bulk homogenous medium.12–14 Although a handful of studies have utilized this three-layer model to analyze in vivo data,12,13,15 the accuracy of this approach is still under investigation.16,17 While it certainly provides a more sophisticated representation of the human head compared to the homogenous model, the three-layer model is still a gross oversimplification. It ignores head curvature, the presence of cerebrospinal fluid (CSF), and heterogeneity in layer thickness. These parameters are important to account for, as they can vary significantly across subjects. Curvature changes dramatically through adolescence as the head circumference undergoes rapid growth. Moreover, the thickness of the CSF layer increases through early adolescence18 and then further increases in old age as the brain begins to shrink.19,20 Finally, layer thickness can be highly heterogenous as a function of optode positioning on the head.18,21–24 Thus, given the high variability in the structure of the human head, a rigorous investigation into the influence of these parameters on estimation accuracy of cerebral blood flow assessed with the three-layer model is needed. In this study, we quantify the influence of head curvature and the CSF layer on the estimation accuracy of cerebral blood flow with the three-layer model. We hypothesize that both of these factors will confound the absolute value of cerebral blood flow index (CBFi) but not the estimation accuracy of relative changes of CBFi (rCBFi). Further, we use Monte Carlo (MC) simulations on MRI head templates spanning a wide age range to demonstrate the cumulative influence of these factors, along with the influence of heterogeneity in layer thickness. Finally, we investigate a pressure modulation process designed to overcome the difficulties of defining layer thickness in vivo. 2.Methods2.1.Influence of CSF2.1.1.Data simulationTo determine the influence of CSF on the estimation of CBFi obtained with the three-layer model, data were simulated with MC eXtreme (MCX)25 on a four-layered slab medium mimicking scalp, skull, CSF, and brain [Fig. 1(a)]. Four separate media were simulated, each of different CSF thickness (1, 2, 3, or 4 mm). All other layer thicknesses, as well as optical properties were constant across simulations: scalp thickness was 6 mm, skull thickness was 6 mm to simulate the frontal region of a healthy young adult,24 and the optical properties (i.e., the absorption and reduced scattering coefficient, and ) of each layer were fixed at , , , , , , , and .19,24,26–29 The anisotropic factor (g) and index of refraction (n) of each layer were fixed at 0.89 and 1.4, respectively. Fig. 1Scheme for investigating the influence of cerebral spinal fluid (CSF) on the accuracy of CBFi with the three-layer model. (a). Data were simulated with MC on a four-slab medium to mimic scalp (blue), skull (yellow), CSF (green), and brain (orange) layers. Simulated data were fit to the three-layer solution of the correlation diffusion equation by assuming CSF belonged either in (b) the second layer () or (c) the third layer (). ![]() For each simulation, photons were injected into the medium. Two 1-mm diameter detectors were placed on the surface of the top (scalp) layer, spaced 1 and 2.5 cm from the source. For each detected photon, MCX records the momentum transfer, scattering angle, and total pathlength traveled in each layer. This information is used to calculate the unnormalized electric field autocorrelation function by assuming a blood flow index in each layer.3 We simulated 12 evenly spaced CBFi values and 6 scalp blood flow index (SBFi) values , for a total of 72 combinations of SBFi and CBFi per simulation. CSF was modeled as a low-scattering medium with negligible () blood flow index.30,31 Similarly, blood flow index of the skull layers was also set to .24 Finally, to simulate experimental measurements, was converted to using the Siegert relation by assuming a coherence factor, , of 0.5.2 2.1.2.Cerebral blood flow estimationSimulated data at 1 and 2.5 cm were simultaneously fit to the three-layer solution of the correlation diffuse equation (CDE) to estimate the blood flow index of the brain and scalp layers (CBFi and SBFi, respectively).16,17 For these fits, optical properties for each layer were assumed to be known and set to the properties listed above. Thickness of the top layer was set to the known thickness of the scalp (6 mm). For the thickness of second layer, we considered two cases. In the first case, CSF was assumed to be part of skull (layer 2), as CSF has negligible blood flow akin to skull. Thus, the thickness of the second layer, , was , where is the thickness of skull and is the thickness of CSF. [Fig. 1(b)]. In the second case, CSF was ignored and lumped with the brain because of its transparency. Thus, the thickness of the second layer was set to that of skull [6 mm, Fig. 1(c)]. In both cases, the blood flow index in the second layer was assumed to be zero. 2.2.Influence of Curvature2.2.1.Data simulationTo determine the influence of head curvature on the estimation of CBFi with the three-layer model, data was simulated using a mesh-based MC32 on a three-layer sphere medium, mimicking scalp, skull, and brain layers. Four separate curvatures were simulated by varying the radius of the outer sphere from 70 to 100 mm in steps of 10 mm.33 These radii were chosen by approximating the head as a circle and extrapolating from normal human head circumference ranges from 1 year to adulthood. Layer thickness and optical properties were constant across simulations and set to the values listed in Sec. 2.1. Detectors were placed along the surface of the outer sphere, spaced 1 and 2.5 cm from the source [ in Fig. 2(a)]. As described in Sec. 2.1, we simulated 72 combinations of SBFi and CBFi for each radius. Fig. 2Scheme for investigating the influence of curvature on the accuracy of with the three-layer model. (a) Data were simulated with MC on a three-layer sphere. denotes the arc distance (green curve) along the surface from the source to the detector while denotes the linear distance. (b) Simulated data were fit to the three-layer solution of the correlation diffusion equation. ![]() 2.2.2.Cerebral blood flow estimationSimulated data were fit to the three-layer solution of the CDE to estimate CBFi and SBFi.16,17 For these fits, optical properties and thickness of each layer were assumed to be known and equal to the true (simulated) value. To confirm the error in estimation of CBFi is caused by the influence of curvature rather than by error in source detector separation (SDS) caused by curvature, we fit data using both the line SDS [ in Fig. 2(a)] and . 2.3.Realistic Human Head Geometry2.3.1.Data simulationTo quantify the accuracy CBFi estimated with the three-layer model in the presence of CSF, head curvature, and heterogeneous layer thicknesses, we simulated data with a mesh-based MC32 on six MRI head templates spanning a wide age range of 5 to 80 years (Fig. 3).34 We limited simulations to years because the homogeneous model has been shown to be sufficient for younger children given the relatively thin extracerebral layers.35,36 Age-averaged MRI templates were obtained from the open-source brain mesh library.37,38 Templates were segmented into scalp, skull, CSF, gray matter, and white matter layers. For simplicity, gray and white matters were lumped together as brain. Optical properties of each layer were assigned as in Sec. 2.1. For these simulations, a source and two detectors were placed over the left forehead in the same axial plane, roughly 1 cm above the eyebrow and spaced and 2.5 cm apart. Detected photons were used to estimate for a range of 72 simulated SBFi and CBFi, as in Sec. 2.1. 2.3.2.Cerebral blood flow estimation with the 3-layer modelSimulated data at 1 and 2.5 cm were simultaneously fit to the three-layer model to estimate CBFi and SBFi.16,17 For these fits, optical properties of each layer were assumed to be known and equal to the true (simulated) value. Layer thickness for these simulations was challenging to determine because of heterogeneities under the region spanned by the optodes. Thus, we explored two approaches to assess the layer thicknesses.
2.4.Evaluations of Estimation Accuracy of CBFiWe calculated the percentage error in estimated CBFi as . Further, because we are often clinically interested in assessing relative changes in CBF as a function of time, we quantified relative changes in CBFi. For each simulated CBFi value, we defined the relative change in CBFi as , where the subscript 0 denotes a baseline/reference measurement, which was arbitrarily chosen to be and . This baseline ensured a wide range of simulated rCBFi ( to 74%) and rSBFi ( to 189%). Error in estimated rCBFi was defined as . 3.Results3.1.Influence of Cerebrospinal FluidGrouping CSF into the second layer leads to large errors in the estimation of CBFi [Fig. 4(a), green]. As CSF thickness increases, CBFi is significantly overestimated. Note, when CSF thickness was 4 mm, the fitting process failed to converge. In contrast, ignoring CSF, i.e., grouping CSF with the third layer, leads to underestimation in CBFi [Fig. 4(a), orange]. In this situation, as the thickness of CSF increases, the error in CBFi decreases monotonically, although the magnitude of this error is considerably less that the case where CSF is grouped with the skull layer. Relative changes in CBFi can be accurately recovered in both models to within approximately 10%; however, the range of error in estimated rCBFi across all combinations of CBFi and SBFi tested is smaller when CSF is grouped in the third layer compared to the second layer [Fig. 4(b)]. Fig. 4Influence of CSF. Boxplots showing the percentage error in estimated CBFi (a) and relative changes in CBFi (rCBFi) (b) when considering CSF as part of second (green) or third (orange) layer during the fitting process. For each boxplot, the central line denotes the median, and the bottom and top edges of the box indicate the 25’th and 75’th percentiles, respectively, of the 72 samples tested. The whiskers extend to the most extreme data points not considered outliers. ![]() 3.2.Influence of Head CurvatureAcross all simulated head circumferences, curvature led to a median underestimation of CBFi of roughly to [Fig. 5(a)]. This error was relatively independent of circumference. Similarly, the choice of source–detector separation (line versus curve) had minimal influence on this underestimation [orange versus green, Fig. 5(a)]. Moreover, errors in rCBFi caused by curvature were within 15% of the true value across all SBFi/CBFi combinations tested [Fig. 5(b)]. Fig. 5Influence of curvature. Boxplots of the percentage error in estimated CBFi (a) and relative changes in CBFi (rCBFi) (b) when using the three-layer analytical model with the arc distance along the surface from the source to the detector (green) or the linear distance between source and detector (orange). For each boxplot, the central line denotes the median, and the bottom and top edges of the box indicate the 25’th and 75’th percentiles, respectively, of the 72 samples tested. The whiskers extend to the most extreme data points not considered outliers. ![]() 3.3.Accuracy of Three-Layer Model as a Function of AgeThe volume-averaged measured thickness of the extracerebral layers in the MRI age-averaged templates increased with age, as expected [Fig. 6(a)]. These increases were driven by increases in skull thickness from 5 to 20 years, and by increases in CSF thickness from 40 to 80 years. Average head circumference sharply increased during adolescence and remained constant throughout adulthood. The standard deviation in the head circumference of 10 to 15 mm reflects the variation of head size across axial slices. Figure 6(b) shows the optimized thickness of the skull and scalp layers derived from the pressure modulation method outlined in Sec. 2.3. As shown in Fig. 6(c), the optimized scalp thickness is correlated with the volume-averaged measured scalp thickness, but the two measures can deviate by as much as 2 mm. In general, the optimized skull thicknesses deviate more from the volume-averaged skull thickness than the optimized/measured scalp thicknesses. Fig. 6(a) Mean and standard deviation of the volume-averaged scalp (dark blue), skull (light blue), and CSF (yellow) thickness as well as head circumference (orange) across several axial slices near the source–detector plane on each MRI templates. (b) Optimized scalp and skull (light and dark blue, respectively) thickness obtained from pressure modulation. (c) Comparison of measured volume-averaged layer thickness with the optimized thickness estimate from pressure modulation for scalp (, in dark blue) and skull (, in light blue). Different shapes denote the six different MRI templates: circle is 5y, diamond is 8y, square is 20y, upper triangle is 40y, right triangle is 70y, and pentagram is 80y. Dotted line denotes the line of unity. ![]() As shown in Fig. 7(a), when using either the homogeneous or the 3-layer model to estimate CBFi from the MRI age-averaged templates, CBFi was typically significantly underestimated. The magnitude of this underestimation varied wildly as a function of age for the three-layer estimations, presumably due to heterogeneity in layer thickness that can have appreciable influence on estimation accuracy. For relative changes in CBFi, the median error across all simulated combinations of CBFi/SBFi was approximately zero. As expected, the variation in this error about the median was appreciably larger for the semi-infinite compared to the three-layer model. The three-layer model with optimized thickness has the smallest range of error in rCBFi across the CBFi/SBFi combinations tested [Fig. 7(b)]. Fig. 7Comparison of three-layer and homogeneous models. Boxplots of the error in estimated CBFi (a) and relative change in CBFi (b) as a function of age. For each boxplot, the central line denotes the median, and the bottom and top edges of the box indicate the 25’th and 75’th percentiles, respectively, of the 72 samples tested. The whiskers extend to the most extreme data points not considered outliers. ![]() 4.DiscussionThe three-layer model is designed to separate cerebral hemodynamics from extracerebral contaminations to the DCS measured signal. Although this model does not fully recapitulate the complex structure of the human head, it strikes a balance between model complexity and estimation accuracy, while providing a significant improvement over the traditional homogenous model, which suffers from substantial extracerebral contaminations. Despite these advantages, there are several key features that the model fails to incorporate, including CSF, head curvature, and layer heterogeneity. The findings herein demonstrate that these factors can lead to appreciable errors in the estimation of cerebral blood flow. However, relative changes in CBFi can be recovered in a manner that is relatively insensitive to these factors, suggesting that the three-layer model is a promising approach to improve brain sensitivity of relative changes in perfusion with DCS. Our results show that grouping CSF with the skull layer [Fig. 1(b)] leads to an overestimation of CBFi, while totally ignoring CSF [Fig. 1(c)] causes an underestimation of CBFi. In general, the correlation diffusion equation (CDE) breaks down in the presence of the very low absorption and scattering coefficient of the CSF. This breakdown can be visualized in Fig. 8 wherein we compare from a three-layered medium with a CSF as layer 2. Data were generated with MC and the three-layer solution to the CDE. The CDE generated curve is right shifted compared to the MC data. Thus, when CSF is grouped with the skull layer as layer 2 [Fig. 1(b)], using the CDE to fit MC-generated data in the presence of CSF leads to an overestimation of CBFi. However, the same overestimation was not found when CSF is grouped with the layer 3 [Fig. 1(c)]. We believe this contrary finding is because in the latter case, other factors also contribute to the error in CBFi. For one, the effective layer 3 flow in this case is a weighted combination of the zero flow CSF and brain flow, so we expect the estimated CBFi to be underestimated. Further, the effective optical properties of layer 3, which are a weighted combination of CSF and brain optical properties, are smaller than the values assumed in the fitting process. Overestimated layer 3 optical properties can have an appreciable effect on CBFi.16 In total, it appears these effects combine to lead to a net underestimation of CBFi, which agrees with a previous work investigating the influence of CSF with the two-layer model.39 We note that the influence of overestimated optical properties is not relevant in the former case when CSF is grouped in layer 2 because errors in layer 2 optical properties have minimal influence on CBFi estimation with the three-layer model.16 Fig. 8Effect of CSF. Comparison of intensity autocorrelation curves, , simulated using Monte Carlo (MC, black) and the correlation diffusion equation (CDE, red) at 1 cm (solid line) and 2.5 cm (dashed line) for a three-layer slab medium consisting of 10 mm scalp/skull (layer 1), 2 mm CSF (layer 2), and brain (layer 3). ![]() Our results also show that curvature can lead to an underestimation of CBFi. Curvature can cause changes in the photon pathlength distribution,40 particularly for photons detected at 2.5 cm [Figs. 9(a) and 9(b)]. These changes are largely caused by a right-shift in the pathlength distribution within the scalp/skull and a slight left shift in the pathlength distribution in the brain [Fig. 9(c)]. The net effect of this distribution change is that the autocorrelation curves are slightly right shifted, which translates to an underestimation of CBFi. As was the case with CSF, these underestimations do not propagate to the error in rCBFi, thus the effect of curvature on rCBFi is minimal. Fig. 9Distribution of the total pathlength of all detected photons at 1 cm (a) and 2.5 cm (b) for simulations performed on the sphere with radius 70 mm. (c) Distribution of pathlength of all detected photons at 2.5 cm in each layer (scalp in gray, skull in yellow, and brain in orange). In each subplot, the solid line denotes the three-layer slab and the dotted line denotes the three-layer sphere geometry. ![]() The simulations performed on the three-/and four-layer slab and sphere models allowed us to isolate the effects of curvature and CSF. In contrast, our modeling results from the MRI age-averaged templates provide insights into the cumulative effects of these factors on the accuracy of CBFi. Consistent with the results from Figs. 4 and 5, we found that CBFi was underestimated in all MRI-templates with the exception of 40 years. As shown in Fig. 10, the magnitude of these errors is highly influenced by small variations in the positions of the source and detector optodes. By varying the axial location of the optodes by , the error in CBFi varied from to 50% [Fig. 10(e)], demonstrating that the cumulative effects of curvature, CSF, and layer heterogeneity on the estimation accuracy of CBFi can be appreciable. We note that the investigation of optode position (Fig. 10) was performed in the 40Y template. We anticipate that as extracerebral thickness decreases, brain sensitivity of the model should increase, and as such, CBFi variability with optode positioning should be reduced. Fig. 10(a) Three different source–detector pair locations (A, B, C) on the surface of the forehead. (b) Visualization of the axial slices containing the source–detector pairs from panel (a). (c) Measured volume-averaged layer thicknesses (scalp in dark blue, skull in light blue, and CSF in yellow) and (d) optimized layer thickness for the three different locations of source–detector pairs shown in (a, b). (e) Boxplots of the error in estimated CBFi and (f) relative change in CBFi (rCBFi) at these locations using the three-layer model with measured thickness (green), optimized thickness (orange), and the homogeneous model (purple). For each boxplot, the central line denotes the median, and the bottom and top edges of the box indicate the 25’th and 75’th percentiles, respectively, of the 72 samples tested. The whiskers extend to the most extreme data points not considered outliers. ![]() To mitigate the errors in CBFi estimation due to the difficulty in defining layer thickness, we investigated an approach to identify an equivalent, “optimized” thickness via a pressure modulation. While this approach did not improve estimation accuracy of CBFi [Figs. 7(a) and 10(e)], it did significantly improve the estimation accuracy of rCBFi, as was suggested by Ref. 13. Thus, we recommend utilizing this pressure modulation approach to improve estimation accuracy of rCBFi when using the three-layer model. However, we note that pressure modulation in vivo may induce variations in scalp thickness that were not accounted for here and that could affect estimation accuracy in practice. Future research is needed to explore the validity of this pressure-modulation approach via an in vivo comparison against a gold standard perfusion technique. There are several limitations in this study. Noise was not considered in our simulations; thus, the errors reported likely represent the best-case scenario. Moreover, we assumed optical properties for scalp, skull, and brain layers were known. Inaccuracies in these properties will likely compound estimation inaccuracies.16 5.ConclusionWe quantified the influence of curvature, CSF, and layer heterogeneity on the estimation of cerebral blood flow when using a three-layer model to analyze DCS data. These factors cause significant errors in CBFi; however, the influence of these factors on the estimation of relative changes in cerebral blood flow are minimal. AcknowledgmentsWe would like to acknowledge the help on MC simulation from Dr. Qianqian Fang and Dr. Shijie Yan. We also would like to acknowledge the help from Kyle R. Cowdrick on IT support. This work is funded by the National Institutes of Health (Grant No. R01‐HL152322). Data and Code Availability StatementThe code and data used to generate figures is available at Github https://github.gatech.edu/Buckley-Lab-Code-Repository/Oversimplification_of_human_head.git. ReferencesT. Durduran and A. G. Yodh,
“Diffuse correlation spectroscopy for non-invasive, micro-vascular cerebral blood flow measurement,”
Neuroimage, 85 51
–63 https://doi.org/10.1016/j.neuroimage.2013.06.017 NEIMEF 1053-8119
(2014).
Google Scholar
T. Durduran et al.,
“Diffuse optics for tissue monitoring and tomography,”
Rep. Prog. Phys., 73
(7), 076701 https://doi.org/10.1088/0034-4885/73/7/076701 RPPHAG 0034-4885
(2010).
Google Scholar
D. A. Boas et al.,
“Establishing the diffuse correlation spectroscopy signal relationship with blood flow,”
Proc. SPIE, 3
(3), 031412 https://doi.org/10.1117/1.nph.3.3.031412 PSISDG 0277-786X
(2016).
Google Scholar
D. A. Boas and A. G. Yodh,
“Spatially varying dynamical properties of turbid media probed with diffusing temporal light correlation,”
J. Opt. Soc. Am., 14
(1), 192 https://doi.org/10.1364/JOSAA.14.000192 JOSAAH 0030-3941
(1997).
Google Scholar
J. Sutin et al.,
“Time-domain diffuse correlation spectroscopy,”
Optica, 3
(9), 1006 https://doi.org/10.1364/OPTICA.3.001006
(2016).
Google Scholar
O. Kholiqov et al.,
“Time-of-flight resolved light field fluctuations reveal deep human tissue physiology,”
Nat. Commun., 11 391 https://doi.org/10.1038/s41467-019-14228-5 NCAOBW 2041-1723
(2020).
Google Scholar
M. B. Robinson et al.,
“Interferometric diffuse correlation spectroscopy improves measurements at long source–detector separation and low photon count rate,”
J. Biomed. Opt., 25
(9), 097004 https://doi.org/10.1117/1.JBO.25.9.097004 JBOPFO 1083-3668
(2020).
Google Scholar
W. Zhou et al.,
“Functional interferometric diffusing wave spectroscopy of the human brain,”
Sci. Adv., 7
(20), eabe0150 https://doi.org/10.1126/sciadv.abe0150 STAMCV 1468-6996
(2021).
Google Scholar
S. A. Carp et al.,
“Diffuse correlation spectroscopy measurements of blood flow using 1064 nm light,”
J. Biomed. Opt., 25
(9), 097003 https://doi.org/10.1117/1.JBO.25.9.097003 JBOPFO 1083-3668
(2020).
Google Scholar
W. B. Baker et al.,
“Modified Beer-Lambert law for blood flow,”
Biomed. Opt. Express, 5
(11), 4053
–4075 https://doi.org/10.1364/BOE.5.004053 BOEICL 2156-7085
(2014).
Google Scholar
W. B. Baker et al.,
“Pressure modulation algorithm to separate cerebral hemodynamic signals from extracerebral artifacts,”
Neurophotonics, 2
(3), 035004 https://doi.org/10.1117/1.NPh.2.3.035004 PSISDG 0277-786X
(2015).
Google Scholar
K. Verdecchia et al.,
“Assessment of a multi-layered diffuse correlation spectroscopy method for monitoring cerebral blood flow in adults,”
Biomed. Opt. Express, 7
(9), 3659 https://doi.org/10.1364/BOE.7.003659 BOEICL 2156-7085
(2016).
Google Scholar
M. M. Wu et al.,
“Improved accuracy of cerebral blood flow quantification in the presence of systemic physiology cross-talk using multi-layer Monte Carlo modeling,”
Neurophotonics, 8
(1), 015001 https://doi.org/10.1117/1.NPh.8.1.015001 PSISDG 0277-786X
(2021).
Google Scholar
J. Li et al.,
“Noninvasive detection of functional brain activity with near-infrared diffusing-wave spectroscopy,”
J. Biomed. Opt., 10
(4), 044002 https://doi.org/10.1117/1.2007987 JBOPFO 1083-3668
(2005).
Google Scholar
D. Milej et al.,
“Direct assessment of extracerebral signal contamination on optical measurements of cerebral blood flow, oxygenation, and metabolism,”
Neurophotonics, 7
(4), 045002 https://doi.org/10.1117/1.NPh.7.4.045002
(2020).
Google Scholar
H. Zhao, E. Sathialingam and E. M. Buckley,
“Accuracy of diffuse correlation spectroscopy measurements of cerebral blood flow when using a three-layer analytical model,”
Biomed. Opt. Express, 12
(11), 7149 https://doi.org/10.1364/BOE.438303 BOEICL 2156-7085
(2021).
Google Scholar
H. Zhao and E. M. Buckley,
“Influence of source–detector separation on diffuse correlation spectroscopy measurements of cerebral blood flow with a multilayered analytical model,”
Neurophotonics, 9
(3), 035002 https://doi.org/10.1117/1.NPh.9.3.035002 PSISDG 0277-786X
(2022).
Google Scholar
M. S. Beauchamp et al.,
“The developmental trajectory of brain-scalp distance from birth through childhood: implications for functional neuroimaging,”
PLoS One, 6
(9), e24981 https://doi.org/10.1371/journal.pone.0024981 POLNCL 1932-6203
(2011).
Google Scholar
D. Ancora et al.,
“Noninvasive optical estimation of CSF thickness for brain-atrophy monitoring,”
Biomed. Opt. Express, 9
(9), 4094
–4112 https://doi.org/10.1364/BOE.9.004094 BOEICL 2156-7085
(2018).
Google Scholar
P. T. Fillmore, M. C. Phillips-Meek and J. E. Richards,
“Age-specific MRI brain and head templates for healthy adults from 20 through 89 years of age,”
Front. Aging Neurosci., 7 44 https://doi.org/10.3389/fnagi.2015.00044
(2015).
Google Scholar
N. J. Davis,
“Variance in cortical depth across the brain surface: implications for transcranial stimulation of the brain,”
Eur. J. Neurosci., 53
(4), 996
–1007 https://doi.org/10.1111/ejn.14957 EJONEI 0953-816X
(2021).
Google Scholar
K. Im et al.,
“Brain size and cortical structure in the adult human brain,”
Cereb. Cortex, 18
(9), 2181
–2191 https://doi.org/10.1093/cercor/bhm244 53OPAV 1047-3211
(2008).
Google Scholar
M. G. Stokes et al.,
“Simple metric for scaling motor threshold based on scalp-cortex distance: application to studies using transcranial magnetic stimulation,”
J. Neurophysiol., 94
(6), 4520
–4527 https://doi.org/10.1152/jn.00067.2005 JONEA4 0022-3077
(2005).
Google Scholar
M. M. Wu et al.,
“Complete head cerebral sensitivity mapping for diffuse correlation spectroscopy using subject-specific magnetic resonance imaging models,”
Biomed. Opt. Express, 13
(3), 1131 https://doi.org/10.1364/BOE.449046 BOEICL 2156-7085
(2022).
Google Scholar
Q. Fang and D. A. Boas,
“Monte Carlo simulation of photon migration in 3D turbid media accelerated by graphics processing units,”
Opt. Express, 17
(22), 20178 https://doi.org/10.1364/OE.17.020178 OPEXFF 1094-4087
(2009).
Google Scholar
A. P. Tran, S. Yan and Q. Fang,
“Improving model-based fNIRS analysis using mesh-based anatomical and light-transport models,”
(2020). Google Scholar
A. Custo et al.,
“Effective scattering coefficient of the cerebral spinal fluid in adult head models for diffuse optical imaging,”
Appl. Opt., 45
(19), 4747 https://doi.org/10.1364/AO.45.004747 APOPAI 0003-6935
(2006).
Google Scholar
G. E. Strangman, Q. Zhang and Z. Li,
“Scalp and skull influence on near infrared photon propagation in the Colin27 brain template,”
Neuroimage, 85 136
–149 https://doi.org/10.1016/j.neuroimage.2013.04.090 NEIMEF 1053-8119
(2014).
Google Scholar
S. Lohrengel et al.,
“A homogenized cerebrospinal fluid model for diffuse optical tomography in the neonatal head,”
Int. J. Numer. Method Biomed. Eng., 38
(1), e3538 https://doi.org/10.1002/cnm.3538
(2021).
Google Scholar
E. Okada and D. T. Delpy,
“Near-infrared light propagation in an adult head model II Effect of superficial tissue thickness on the sensitivity of the near-infrared spectroscopy signal,”
Appl. Opt., 42
(16), 2915 https://doi.org/10.1364/AO.42.002915 APOPAI 0003-6935
(2003).
Google Scholar
E. Okada and D. T. Delpy,
“Near-infrared light propagation in an adult head model I modeling of low-level scattering in the cerebrospinal fluid layer,”
Appl. Opt., 42
(16), 2906 https://doi.org/10.1364/AO.42.002906 APOPAI 0003-6935
(2003).
Google Scholar
S. Yan, A. P. Tran and Q. Fang,
“Dual-grid mesh-based Monte Carlo algorithm for efficient photon transport simulations in complex three-dimensional media,”
J. Biomed. Opt., 24
(2), 020503 https://doi.org/10.1117/1.JBO.24.2.020503 JBOPFO 1083-3668
(2019).
Google Scholar
J. D. Rollins, J. S. Collins and K. R. Holden,
“United States head circumference growth reference charts: birth to 21 years,”
J. Pediatr., 156
(6), 907
–913.e2 https://doi.org/10.1016/j.jpeds.2010.01.009
(2010).
Google Scholar
Y. Yuan et al.,
“Transcranial photobiomodulation with near-infrared light from childhood to elderliness: simulation of dosimetry,”
Neurophotonics, 7
(1), 015009 https://doi.org/10.1117/1.NPh.7.1.015009 PSISDG 0277-786X
(2020).
Google Scholar
V. Jain et al.,
“Cerebral oxygen metabolism in neonates with congenital heart disease quantified by MRI and optics,”
J. Cereb. Blood Flow Metab., 34
(3), 380
–388 https://doi.org/10.1038/jcbfm.2013.214
(2013).
Google Scholar
E. M. Buckley et al.,
“Validation of diffuse correlation spectroscopic measurement of cerebral blood flow using phase-encoded velocity mapping magnetic resonance imaging,”
J. Biomed. Opt., 17
(3), 037007 https://doi.org/10.1117/1.JBO.17.3.037007 JBOPFO 1083-3668
(2012).
Google Scholar
J. E. Richards et al.,
“A database of age-appropriate average MRI templates,”
Neuroimage, 124
(Pt B), 1254
–1259 https://doi.org/10.1016/j.neuroimage.2015.04.055 NEIMEF 1053-8119
(2016).
Google Scholar
A. P. Tran, S. Yan and Q. Fang,
“Improving model-based functional near-infrared spectroscopy analysis using mesh-based anatomical and light-transport models,”
Neurophotonics, 7
(1), 015008 https://doi.org/10.1117/1.NPh.7.1.015008 PSISDG 0277-786X
(2020).
Google Scholar
F. Jaillon et al.,
“Diffusing-wave spectroscopy from head-like tissue phantoms: influence of a non-scattering layer,”
Opt. Express, 14
(22), 10181 https://doi.org/10.1364/OE.14.010181 OPEXFF 1094-4087
(2006).
Google Scholar
D. Piao et al.,
“On the geometry dependence of differential pathlength factor for near-infrared spectroscopy. I. Steady-state with homogeneous medium,”
J. Biomed. Opt., 20
(10), 105005 https://doi.org/10.1117/1.JBO.20.10.105005 JBOPFO 1083-3668
(2015).
Google Scholar
BiographyHongting Zhao is a PhD student in the Wallace H. Coulter Department of Biomedical Engineering at Georgia Institute of Technology and Emory University. Her research interests include improving estimation accuracy of cerebral blood flow using diffuse correlation spectroscopy. Erin M. Buckley is an assistant professor in the Wallace H. Coulter Department of Biomedical Engineering at Georgia Institute of Technology and Emory University, and the Department of Pediatrics at Emory University. Her research interest includes the development of translational diffuse optical spectroscopies for noninvasive low-cost bedside monitoring of hemodynamics in brain and other tissues. |
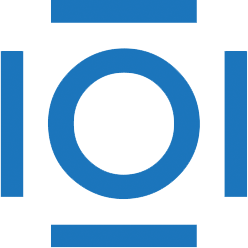
CITATIONS
Cited by 5 scholarly publications.
Head
Skull
Cerebral blood flow
Data modeling
Error analysis
Brain
Analytic models