|
ACCESS THE FULL ARTICLE
No SPIE Account? Create one
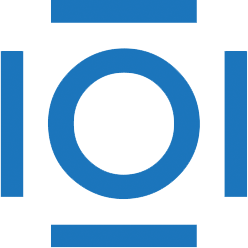
CITATIONS
Cited by 1 scholarly publication.
Convolution
Hyperspectral imaging
Image classification
Principal component analysis
3D modeling
Feature extraction
Convolutional neural networks