|
ACCESS THE FULL ARTICLE
No SPIE Account? Create one
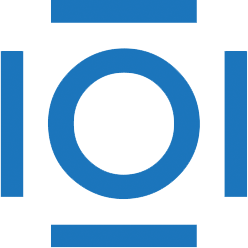
CITATIONS
Cited by 11 scholarly publications.
Mammography
Breast cancer
Cancer
Data centers
Neural networks
Iterative methods
Virtual colonoscopy