Methods: The main contribution is to estimate the deformation between prostate MRI and CT images in a patch-wise fashion by using the sparse representation technique. We assume that two image patches should follow the same deformation if their patch-wise appearance patterns are similar. Specifically, there are two stages in our proposed framework, i.e., the training stage and the application stage. In the training stage, each prostate MR images are carefully registered to the corresponding CT images and all training MR and CT images are carefully registered to a selected CT template. Thus, we obtain the dense deformation field for each training MR and CT image. In the application stage, for registering a new subject MR image with the same subject CT image, we first select a small number of key points at the distinctive regions of this subject CT image. Then, for each key point in the subject CT image, we extract the image patch, centered at the underlying key point. Then, we adaptively construct the coupled dictionary for the underlying point where each atom in the dictionary consists of image patches and the respective deformations obtained from training pair-wise MRI-CT images. Next, the subject image patch can be sparsely represented by a linear combination of training image patches in the dictionary, where we apply the same sparse coefficients to the respective deformations in the dictionary to predict the deformation for the subject MR image patch. After we repeat the same procedure for each subject CT key point, we use B-splines to interpolate a dense deformation field, which is used as the initialization to allow the registration algorithm estimating the remaining small segment of deformations from MRI to CT image. Results: Our MRI-CT registration technique was validated with a clinical study of 10 patients. The accuracy of our approach was assessed using some identified landmarks in both MRI and CT images. Our proposed registration was compared with the current free-form-deformation (FFD)-based registration method. The accuracy of the proposed method was significantly higher than the commonly used FFD-based registration utilizing normalized mutual information (NMI). Conclusions: We have developed a new prostate MR-CT registration approach based on patch-deformation dictionary, demonstrated its clinical feasibility, and validated its accuracy with some identified landmarks. The proposed registration method may provide an accurate and robust means of estimating prostate-gland deformation between MRI and CT scans, and is therefore well-suited for a number of MR-targeted CT-based prostate radiotherapy. |
ACCESS THE FULL ARTICLE
No SPIE Account? Create one
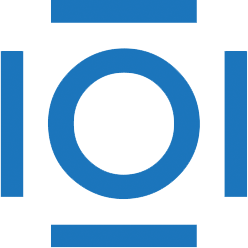
CITATIONS
Cited by 3 scholarly publications.
Computed tomography
Image registration
Magnetic resonance imaging
Prostate
Associative arrays
Associative arrays
Radiotherapy