|
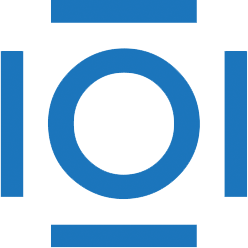
CITATIONS
Cited by 51 scholarly publications and 4 patents.
Lung
Computer aided diagnosis and therapy
Feature extraction
Lung cancer
Computed tomography
Computer aided design
Diagnostics